-
Notifications
You must be signed in to change notification settings - Fork 89
Commit
This commit does not belong to any branch on this repository, and may belong to a fork outside of the repository.
- Loading branch information
Showing
5 changed files
with
202 additions
and
2 deletions.
There are no files selected for viewing
This file contains bidirectional Unicode text that may be interpreted or compiled differently than what appears below. To review, open the file in an editor that reveals hidden Unicode characters.
Learn more about bidirectional Unicode characters
Binary file not shown.
187 changes: 187 additions & 0 deletions
187
open-machine-learning-jupyter-book/deep-learning/rnn/bi-rnn.ipynb
This file contains bidirectional Unicode text that may be interpreted or compiled differently than what appears below. To review, open the file in an editor that reveals hidden Unicode characters.
Learn more about bidirectional Unicode characters
Original file line number | Diff line number | Diff line change |
---|---|---|
@@ -0,0 +1,187 @@ | ||
{ | ||
"cells": [ | ||
{ | ||
"cell_type": "markdown", | ||
"metadata": {}, | ||
"source": [ | ||
"# Bidirectional RNN\n", | ||
"\n", | ||
"We already know about LSTM in RNN, how can we further optimize a model like RNN? That's using Bidirectional RNN.\n", | ||
"\n", | ||
"In 1997, Mike Schuster proposed the Bidirectional RNN model. These two models greatly improve the early RNN structure, broaden the application range of RNN, and lay a foundation for the development of subsequent sequence modeling." | ||
] | ||
}, | ||
{ | ||
"cell_type": "markdown", | ||
"metadata": {}, | ||
"source": [ | ||
"## Overview\n", | ||
"In the RNN, only the word before the prediction word is considered, that is, only the \"above\" in the context is considered, and the content after the word is not considered. This may miss some important information, making the content of the forecast less accurate. As in the case of TV shows, when a new character is introduced in the episode, the name of that character cannot be effectively predicted based on the content of previous episodes. But if we watch the later episodes, we might be able to make more effective predictions. Bidirectional RNNS are also based on the idea that they not only keep important information about the words before the word from the front to the back, but also keep important information about the words after the word from the back to the front, and then predict the word based on this important information.\n", | ||
"First, let's see the architecture of BRNN cell." | ||
] | ||
}, | ||
{ | ||
"cell_type": "markdown", | ||
"metadata": {}, | ||
"source": [ | ||
"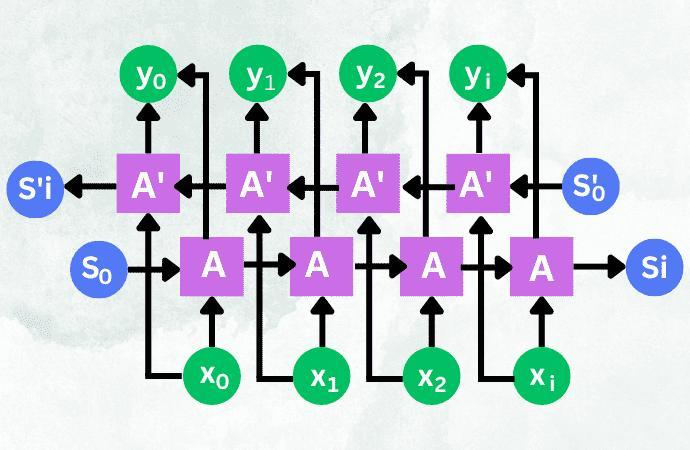 " | ||
] | ||
}, | ||
{ | ||
"cell_type": "markdown", | ||
"metadata": {}, | ||
"source": [ | ||
"### Code\n", | ||
"Here we implement an Bi-RNN model on all a data set of Shakespeare works." | ||
] | ||
}, | ||
{ | ||
"cell_type": "code", | ||
"execution_count": 11, | ||
"metadata": {}, | ||
"outputs": [], | ||
"source": [ | ||
"import tensorflow as tf\n", | ||
"import matplotlib.pyplot as plt\n", | ||
"import numpy as np\n", | ||
"from keras.datasets import imdb\n", | ||
"(x_train, y_train), (x_test, y_test) = imdb.load_data(\"../../assets/data/imdb.npz\", num_words=1000)" | ||
] | ||
}, | ||
{ | ||
"cell_type": "markdown", | ||
"metadata": {}, | ||
"source": [ | ||
"Padding is a technique commonly used in natural language processing (NLP) to ensure that all input sequences have the same length. This is often necessary because many NLP models, such as neural networks, require fixed-length input sequences." | ||
] | ||
}, | ||
{ | ||
"cell_type": "code", | ||
"execution_count": 12, | ||
"metadata": {}, | ||
"outputs": [], | ||
"source": [ | ||
"max_len = 500\n", | ||
"x_train = tf.keras.preprocessing.sequence.pad_sequences(x_train, maxlen=max_len)\n", | ||
"x_test = tf.keras.preprocessing.sequence.pad_sequences(x_test, maxlen=max_len)\n" | ||
] | ||
}, | ||
{ | ||
"cell_type": "markdown", | ||
"metadata": {}, | ||
"source": [ | ||
"Build model" | ||
] | ||
}, | ||
{ | ||
"cell_type": "code", | ||
"execution_count": 13, | ||
"metadata": {}, | ||
"outputs": [], | ||
"source": [ | ||
"input_dim = 10000\n", | ||
"output_dim = 1\n", | ||
"# Create the input layer\n", | ||
"inputs = tf.keras.Input(shape=(None,), dtype=\"int32\")\n", | ||
" \n", | ||
"# Create the model\n", | ||
"x = tf.keras.layers.Embedding(input_dim, 128)(inputs)\n", | ||
"x = tf.keras.layers.Bidirectional(tf.keras.layers.LSTM(64, return_sequences=True))(x)\n", | ||
"x = tf.keras.layers.Bidirectional(tf.keras.layers.LSTM(64))(x)\n", | ||
"outputs = tf.keras.layers.Dense(output_dim, activation=\"sigmoid\")(x)\n", | ||
"model = tf.keras.Model(inputs, outputs)\n", | ||
" \n", | ||
"# Compile the model\n", | ||
"model.compile(\"adam\", \"binary_crossentropy\", metrics=[\"accuracy\"])" | ||
] | ||
}, | ||
{ | ||
"cell_type": "markdown", | ||
"metadata": {}, | ||
"source": [ | ||
"Train the defined model using the imported data" | ||
] | ||
}, | ||
{ | ||
"cell_type": "code", | ||
"execution_count": null, | ||
"metadata": {}, | ||
"outputs": [], | ||
"source": [ | ||
"\n", | ||
"batch_size = 32\n", | ||
"epochs = 5\n", | ||
"history = model.fit(x_train, y_train, batch_size=batch_size, epochs=epochs, validation_data=(x_test, y_test))" | ||
] | ||
}, | ||
{ | ||
"cell_type": "markdown", | ||
"metadata": {}, | ||
"source": [ | ||
"Evaluation accuracy" | ||
] | ||
}, | ||
{ | ||
"cell_type": "code", | ||
"execution_count": null, | ||
"metadata": {}, | ||
"outputs": [], | ||
"source": [ | ||
"\n", | ||
"fig = plt.plot(history.history['accuracy'])\n", | ||
"title = plt.title(\"History\")\n", | ||
"xlabel = plt.xlabel(\"Epochs\")\n", | ||
"ylabel = plt.ylabel(\"Accuracy\")" | ||
] | ||
}, | ||
{ | ||
"cell_type": "markdown", | ||
"metadata": {}, | ||
"source": [ | ||
"forecast" | ||
] | ||
}, | ||
{ | ||
"cell_type": "code", | ||
"execution_count": null, | ||
"metadata": {}, | ||
"outputs": [], | ||
"source": [ | ||
"\n", | ||
"predictions = model.predict(x_test[:4])\n", | ||
"for pred in predictions:\n", | ||
" print(np.argmax(pred[0]))" | ||
] | ||
}, | ||
{ | ||
"cell_type": "markdown", | ||
"metadata": {}, | ||
"source": [ | ||
"## Acknowledgments\n", | ||
"\n", | ||
"Thanks to [Xin Hua](https://blog.csdn.net/mzgxinhua/article/details/135172830).It inspires the majority of the content in this chapter.\n" | ||
] | ||
} | ||
], | ||
"metadata": { | ||
"kernelspec": { | ||
"display_name": "open-machine-learning-jupyter-book", | ||
"language": "python", | ||
"name": "python3" | ||
}, | ||
"language_info": { | ||
"codemirror_mode": { | ||
"name": "ipython", | ||
"version": 3 | ||
}, | ||
"file_extension": ".py", | ||
"mimetype": "text/x-python", | ||
"name": "python", | ||
"nbconvert_exporter": "python", | ||
"pygments_lexer": "ipython3", | ||
"version": "3.9.18" | ||
} | ||
}, | ||
"nbformat": 4, | ||
"nbformat_minor": 2 | ||
} |
File renamed without changes.
This file contains bidirectional Unicode text that may be interpreted or compiled differently than what appears below. To review, open the file in an editor that reveals hidden Unicode characters.
Learn more about bidirectional Unicode characters