-
Notifications
You must be signed in to change notification settings - Fork 3
Commit
This commit does not belong to any branch on this repository, and may belong to a fork outside of the repository.
✍️ Add first post: Creating Gradio-based NL2SQL chatbot
- Loading branch information
Showing
4 changed files
with
173 additions
and
1 deletion.
There are no files selected for viewing
This file contains bidirectional Unicode text that may be interpreted or compiled differently than what appears below. To review, open the file in an editor that reveals hidden Unicode characters.
Learn more about bidirectional Unicode characters
Original file line number | Diff line number | Diff line change |
---|---|---|
@@ -1,6 +1,6 @@ | ||
title: "Hugging Face KREW" | ||
email: [email protected] | ||
timezone: KST | ||
timezone: Asia/Seoul | ||
baseurl: | ||
permalink: "/:title/" | ||
logo: 'assets/images/logo.png' | ||
|
@@ -39,6 +39,13 @@ authors: | |
web: https://www.wowthemes.net/category/jekyll-themes/ | ||
twitter: https://twitter.com/wowthemesnet | ||
description: "This is the author box. Write a short description of the author here. You are currently previewing Affiliates theme demo, a Jekyll template compatible with Github pages." | ||
chaewon: | ||
name: Song Chaewon | ||
display_name: Chaewon | ||
avatar: 'assets/images/author_chaewon.png' | ||
email: [email protected] | ||
web: https://github.com/chhaewxn/ | ||
description: "Hello! I'm Chaewon Song, a developer aspiring to become an Software engineer 🤗" | ||
|
||
# Social | ||
social: | ||
|
165 changes: 165 additions & 0 deletions
165
_posts/2024-09-19-creating-gradio-based-NL2SQL-chatbot.md
This file contains bidirectional Unicode text that may be interpreted or compiled differently than what appears below. To review, open the file in an editor that reveals hidden Unicode characters.
Learn more about bidirectional Unicode characters
Original file line number | Diff line number | Diff line change |
---|---|---|
@@ -0,0 +1,165 @@ | ||
--- | ||
layout: post | ||
title: "Gradio 기반 NL2SQL 챗봇 만들기 - Part 1: 이론과 데이터셋" | ||
author: chaewon | ||
categories: [NLP, SQL, chatbot] | ||
image: assets/images/gradio_free.png | ||
--- | ||
|
||
안녕하세요🤗 첫 포스트는 NL2SQL 챗봇 개발 시리즈의 첫 번째 파트로, 이론적 배경과 데이터셋에 대해 다뤄보려합니다. NL2SQL의 기본 개념, 필요성, 그리고 데이터셋에 대해 자세히 알아보겠습니다. | ||
|
||
이번 포스트는 도서 'LLM을 활용한 실전 AI 애플리케이션 개발 (저자: 허정준)'을 참고하여 작성하였습니다. | ||
|
||
## 1. NL2SQL이란? | ||
|
||
NL2SQL(Natural Language to SQL)은 사용자의 자연어 질문을 SQL(Structured Query Language) 쿼리로 변환하는 기술입니다. 이 기술은 데이터베이스에서 정보를 추출하는 과정을 크게 단순화할 수 있습니다. | ||
|
||
### 1.1 SQL의 중요성 | ||
|
||
SQL은 관계형 데이터베이스에서 데이터를 생성, 조회, 업데이트, 삭제하기 위해 사용되는 표준 언어입니다. 데이터 분석과 관리에 필수적인 도구이지만, 비전문가에게는 학습 곡선이 높을 수 있습니다. | ||
|
||
### 1.2 NL2SQL의 필요성 | ||
|
||
NL2SQL 기술은 다음과 같은 이점을 제공합니다: | ||
|
||
1. **생산성 향상**: 데이터 전문가들의 SQL 작성 시간을 줄여줍니다. | ||
2. **접근성 개선**: SQL을 모르는 비즈니스 사용자들도 데이터베이스에 쉽게 접근할 수 있게 합니다. | ||
3. **오류 감소**: 복잡한 쿼리 작성 시 발생할 수 있는 인적 오류를 줄입니다. | ||
4. **실시간 데이터 분석**: 신속한 의사결정을 위한 즉각적인 데이터 접근을 가능케 합니다. | ||
|
||
## 2. NL2SQL 데이터셋 | ||
|
||
NL2SQL 모델을 훈련시키기 위해서는 적절한 데이터셋이 필요합니다. 여기서는 대표적인 데이터셋과 실습에 사용할 합성 데이터셋에 대해 알아보겠습니다. | ||
|
||
### 2.1 대표적인 NL2SQL 데이터셋 | ||
|
||
#### WikiSQL | ||
|
||
WikiSQL은 단일 테이블에 대한 간단한 쿼리를 생성하는 데 중점을 둔 데이터셋입니다. | ||
|
||
특징: | ||
- 하나의 테이블만 사용 | ||
- SELECT 문에 단일 칼럼 사용 | ||
- 조건절에 최대 3개의 조건만 사용 | ||
|
||
예시: | ||
```json | ||
{ | ||
"phase": 1, | ||
"question": "How would you answer a second test question?", | ||
"sql": { | ||
"agg": 0, | ||
"conds": { | ||
"column_index": [2], | ||
"condition": ["Some Entity"], | ||
"operator_index": [0] | ||
}, | ||
"human_readable": "SELECT Header1 FROM table WHERE Another Header = Some Entity", | ||
"sel": 0 | ||
}, | ||
"table": "{\"caption\": \"L\", \"header\": [\"Header1\", \"Header 2\", \"Another Header\"], \"id\": \"1-10015132-9\", \"name\": \"table_10015132_11\", \"page_i...}" | ||
} | ||
``` | ||
|
||
#### Spider | ||
|
||
Spider는 WikiSQL보다 더 복잡하고 현실적인 시나리오를 다룹니다. | ||
|
||
특징: | ||
- 다중 테이블 지원 | ||
- 복잡한 SQL 구문 포함 (ORDER BY, GROUP BY, HAVING, JOIN 등) | ||
- 다양한 도메인의 데이터베이스 스키마 포함 | ||
|
||
### 2.2 합성 데이터 활용 | ||
|
||
실제 프로젝트에서는 특정 도메인이나 언어에 맞는 데이터셋이 필요할 수 있습니다. 이번 실습에서는 GPT 모델을 활용해 생성한 한국어 NL2SQL 데이터셋을 사용합니다. | ||
|
||
데이터셋 출처: [https://huggingface.co/datasets/shangrilar/ko_text2sql](https://huggingface.co/datasets/shangrilar/ko_text2sql) | ||
|
||
#### 데이터셋 구조 | ||
|
||
| 컬럼 | 설명 | | ||
|------|------| | ||
| db_id | 테이블이 포함된 데이터베이스의 ID | | ||
| context | SQL 생성에 사용할 테이블 정보 | | ||
| question | 데이터 요청사항 | | ||
| answer | 요청에 대한 SQL 정답 | | ||
|
||
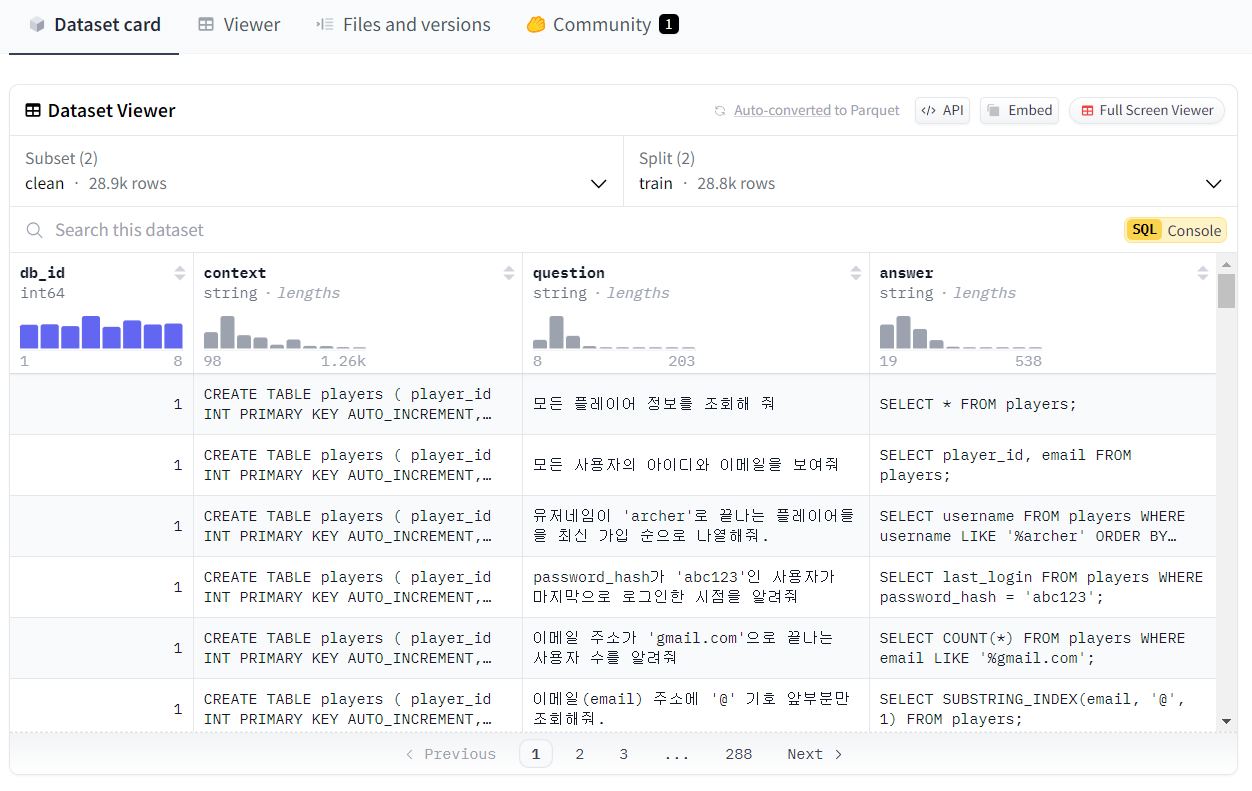 | ||
|
||
## 3. NL2SQL 모델 성능 평가 | ||
|
||
NL2SQL 모델의 성능을 평가하는 것은 매우 중요하지만, 동시에 복잡한 과제입니다. 여기서는 일반적인 평가 방식과 이번 프로젝트에서 사용할 GPT-4 기반 평가 방식에 대해 알아보겠습니다. | ||
|
||
### 3.1 일반적인 평가 방식 | ||
|
||
1. **Exact Match (EM)**: 생성된 SQL 쿼리와 정답 쿼리의 문자열이 정확히 일치하는지 확인합니다. | ||
- 장점: 구현이 간단함 | ||
- 단점: 의미상으로 동일하지만 구문이 다른 쿼리를 틀렸다고 판단할 수 있음 | ||
|
||
2. **Execution Accuracy (EX)**: 생성된 SQL 쿼리를 실제 데이터베이스에서 실행하여 결과가 정답과 일치하는지 확인합니다. | ||
- 장점: 실제 결과를 기반으로 평가하므로 더 정확함 | ||
- 단점: 평가용 데이터베이스 구축이 필요하며, 구현이 복잡함 | ||
|
||
### 3.2 GPT-4를 활용한 평가 방식 | ||
|
||
최근에는 LLM을 활용해 LLM의 생성 결과를 평가하는 방식이 연구되고 있습니다. 이 프로젝트에서는 GPT-4를 사용하여 생성된 SQL과 정답 SQL을 비교 평가합니다. | ||
|
||
#### 평가 프로세스 | ||
|
||
1. 평가 데이터셋 구축 | ||
2. SQL 생성을 위한 프롬프트 준비 | ||
3. GPT-4 평가를 위한 프롬프트와 API 요청 코드 작성 | ||
|
||
#### 프롬프트 예시 | ||
|
||
```python | ||
def make_prompt(ddl, question, query=''): | ||
prompt = f"""당신은 SQL을 생성하는 SQL 봇입니다. DDL의 테이블을 활용한 Question을 해결할 수 있는 SQL 쿼리를 생성하세요. | ||
### DDL: | ||
{ddl} | ||
### Question: | ||
{question} | ||
### SQL: | ||
{query}""" | ||
return prompt | ||
``` | ||
|
||
#### GPT-4 평가 요청 준비 | ||
|
||
```python | ||
def make_requests_for_gpt_evaluation(df, filename, dir='requests'): | ||
if not Path(dir).exists(): | ||
Path(dir).mkdir(parents=True) | ||
prompts = [] | ||
for idx, row in df.iterrows(): | ||
prompts.append("""Based on below DDL and Question, evaluate gen_sql can resolve Question. If gen_sql and gt_sql do equal job, return "yes" else return "no". Output JSON Format: {"resolve_yn": ""}""" + f""" | ||
DDL: {row['context']} | ||
Question: {row['question']} | ||
gt_sql: {row['answer']} | ||
gen_sql: {row['gen_sql']}""" | ||
) | ||
|
||
jobs = [{"model": "gpt-4-turbo-preview", "response_format": { "type": "json_object" }, "messages": [{"role": "system", "content": prompt}]} for prompt in prompts] | ||
with open(Path(dir, filename), "w") as f: | ||
for job in jobs: | ||
json_string = json.dumps(job) | ||
f.write(json_string + "\n") | ||
``` | ||
|
||
## 결론 | ||
|
||
이번 포스트에서는 NL2SQL의 기본 개념, 주요 데이터셋, 그리고 성능 평가 방식에 대해 알아보았습니다. NL2SQL 기술은 데이터베이스 접근성을 크게 향상시키고, 데이터 기반 의사결정을 더욱 효율적으로 만들 수 있습니다. | ||
|
||
다음 포스트에서는 실제 Gradio를 사용하여 NL2SQL 챗봇을 구현하는 방법에 대해 자세히 알아보겠습니다. 궁금한 점이나 의견이 있다면 댓글로 남겨주세요! | ||
|
||
## 참고 자료 | ||
- LLM을 활용한 실전 AI 애플리케이션 개발 (저자: 허정준) | ||
- [WikiSQL 데이터셋](https://huggingface.co/datasets/Salesforce/wikisql) | ||
- [Spider 데이터셋](https://yale-lily.github.io/spider) | ||
- [한국어 NL2SQL 데이터셋](https://huggingface.co/datasets/shangrilar/ko_text2sql) | ||
- [OpenAI API Documentation](https://platform.openai.com/docs/api-reference) |
Loading
Sorry, something went wrong. Reload?
Sorry, we cannot display this file.
Sorry, this file is invalid so it cannot be displayed.
Loading
Sorry, something went wrong. Reload?
Sorry, we cannot display this file.
Sorry, this file is invalid so it cannot be displayed.