diff --git a/docs/source/en/_toctree.yml b/docs/source/en/_toctree.yml
index 58c9b317bc754b..da7ad1fd70c651 100644
--- a/docs/source/en/_toctree.yml
+++ b/docs/source/en/_toctree.yml
@@ -73,6 +73,8 @@
title: Depth estimation
- local: tasks/image_to_image
title: Image-to-Image
+ - local: tasks/mask_generation
+ title: Mask Generation
- local: tasks/knowledge_distillation_for_image_classification
title: Knowledge Distillation for Computer Vision
title: Computer Vision
diff --git a/docs/source/en/tasks/mask_generation.md b/docs/source/en/tasks/mask_generation.md
new file mode 100644
index 00000000000000..e16b014f3757ab
--- /dev/null
+++ b/docs/source/en/tasks/mask_generation.md
@@ -0,0 +1,238 @@
+
+
+# Mask Generation
+
+Mask generation is the task of generating semantically meaningful masks for an image.
+This task is very similar to [image segmentation](semantic_segmentation), but many differences exist. Image segmentation models are trained on labeled datasets and are limited to the classes they have seen during training; they return a set of masks and corresponding classes, given an image.
+
+Mask generation models are trained on large amounts of data and operate in two modes.
+- Prompting mode: In this mode, the model takes in an image and a prompt, where a prompt can be a 2D point location (XY coordinates) in the image within an object or a bounding box surrounding an object. In prompting mode, the model only returns the mask over the object
+that the prompt is pointing out.
+- Segment Everything mode: In segment everything, given an image, the model generates every mask in the image. To do so, a grid of points is generated and overlaid on the image for inference.
+
+Mask generation task is supported by [Segment Anything Model (SAM)](model_doc/sam). It's a powerful model that consists of a Vision Transformer-based image encoder, a prompt encoder, and a two-way transformer mask decoder. Images and prompts are encoded, and the decoder takes these embeddings and generates valid masks.
+
+
+
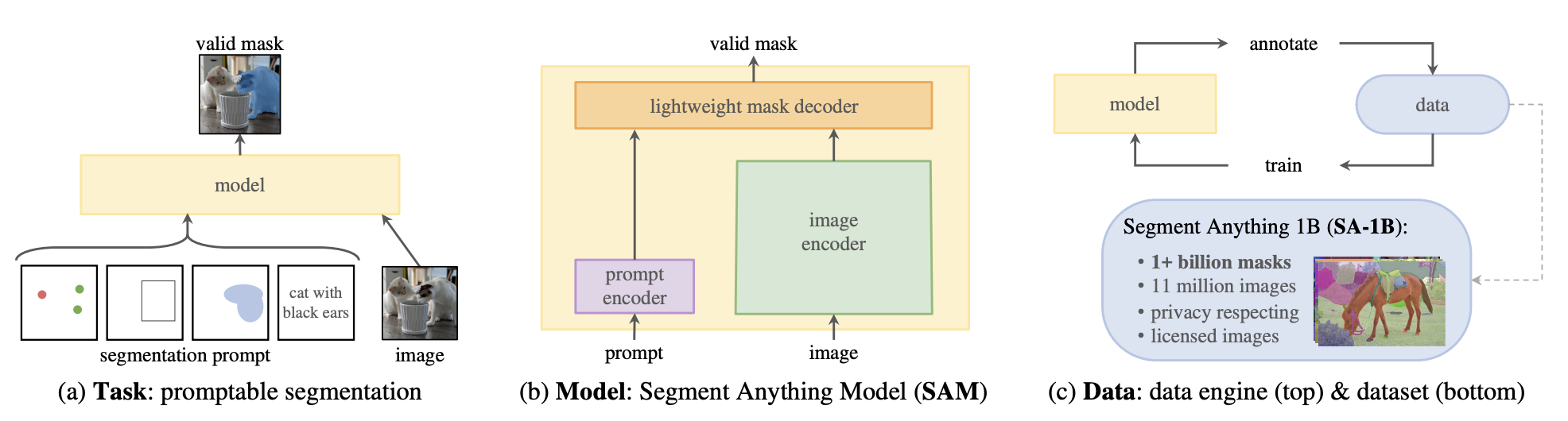
+
+
+SAM serves as a powerful foundation model for segmentation as it has large data coverage. It is trained on
+[SA-1B](https://ai.meta.com/datasets/segment-anything/), a dataset with 1 million images and 1.1 billion masks.
+
+In this guide, you will learn how to:
+- Infer in segment everything mode with batching,
+- Infer in point prompting mode,
+- Infer in box prompting mode.
+
+First, let's install `transformers`:
+
+```bash
+pip install -q transformers
+```
+
+## Mask Generation Pipeline
+
+The easiest way to infer mask generation models is to use the `mask-generation` pipeline.
+
+```python
+>>> from transformers import pipeline
+
+>>> checkpoint = "facebook/sam-vit-base"
+>>> mask_generator = pipeline(model=checkpoint, task="mask-generation")
+```
+
+Let's see the image.
+
+```python
+from PIL import Image
+import requests
+
+img_url = "https://huggingface.co/datasets/huggingface/documentation-images/resolve/main/bee.jpg"
+image = Image.open(requests.get(img_url, stream=True).raw).convert("RGB")
+```
+
+
+
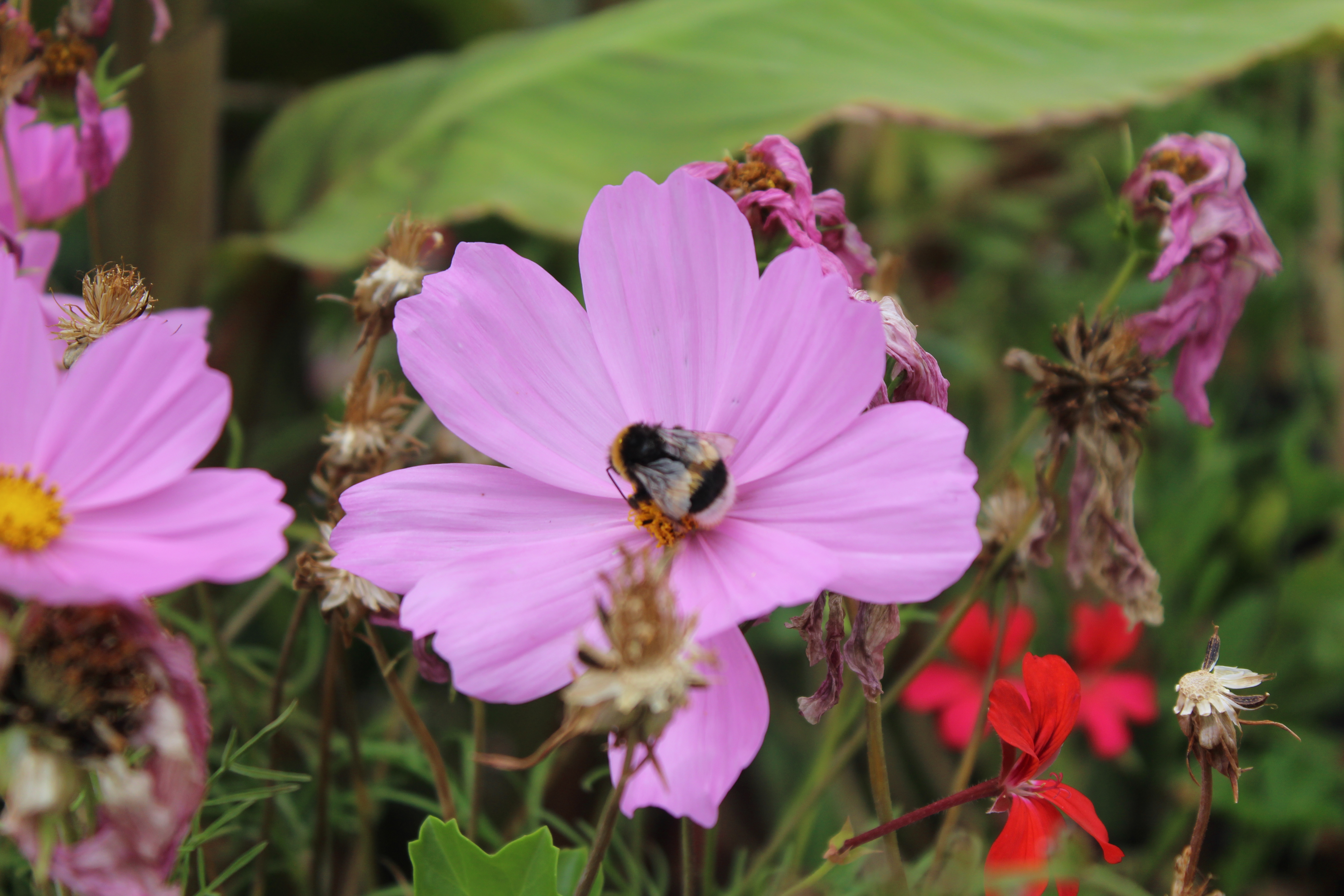
+
+
+Let's segment everything. `points-per-batch` enables parallel inference of points in segment everything mode. This enables faster inference, but consumes more memory. Moreover, SAM only enables batching over points and not the images. `pred_iou_thresh` is the IoU confidence threshold where only the masks above that certain threshold are returned.
+
+```python
+masks = mask_generator(image, points_per_batch=128, pred_iou_thresh=0.88)
+```
+
+The `masks` looks like the following:
+
+```bash
+{'masks': [array([[False, False, False, ..., True, True, True],
+ [False, False, False, ..., True, True, True],
+ [False, False, False, ..., True, True, True],
+ ...,
+ [False, False, False, ..., False, False, False],
+ [False, False, False, ..., False, False, False],
+ [False, False, False, ..., False, False, False]]),
+ array([[False, False, False, ..., False, False, False],
+ [False, False, False, ..., False, False, False],
+ [False, False, False, ..., False, False, False],
+ ...,
+'scores': tensor([0.9972, 0.9917,
+ ...,
+}
+```
+
+We can visualize them like this:
+
+```python
+import matplotlib.pyplot as plt
+
+plt.imshow(image, cmap='gray')
+
+for i, mask in enumerate(masks["masks"]):
+ plt.imshow(mask, cmap='viridis', alpha=0.1, vmin=0, vmax=1)
+
+plt.axis('off')
+plt.show()
+```
+
+Below is the original image in grayscale with colorful maps overlaid. Very impressive.
+
+
+
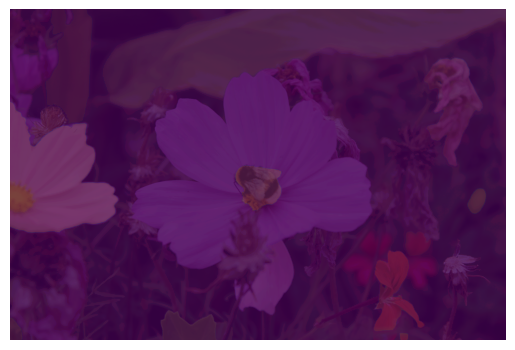
+
+
+
+## Model Inference
+
+### Point Prompting
+
+You can also use the model without the pipeline. To do so, initialize the model and
+the processor.
+
+```python
+from transformers import SamModel, SamProcessor
+
+device = torch.device('cuda' if torch.cuda.is_available() else 'cpu')
+
+model = SamModel.from_pretrained("facebook/sam-vit-base").to(device)
+processor = SamProcessor.from_pretrained("facebook/sam-vit-base")
+```
+
+To do point prompting, pass the input point to the processor, then take the processor output
+and pass it to the model for inference. To post-process the model output, pass the outputs and
+`original_sizes` and `reshaped_input_sizes` we take from the processor's initial output. We need to pass these
+since the processor resizes the image, and the output needs to be extrapolated.
+
+```python
+input_points = [[[2592, 1728]]] # point location of the bee
+
+inputs = processor(image, input_points=input_points, return_tensors="pt").to(device)
+with torch.no_grad():
+ outputs = model(**inputs)
+masks = processor.image_processor.post_process_masks(outputs.pred_masks.cpu(), inputs["original_sizes"].cpu(), inputs["reshaped_input_sizes"].cpu())
+```
+We can visualize the three masks in the `masks` output.
+
+```python
+import torch
+import matplotlib.pyplot as plt
+import numpy as np
+
+fig, axes = plt.subplots(1, 4, figsize=(15, 5))
+
+axes[0].imshow(image)
+axes[0].set_title('Original Image')
+mask_list = [masks[0][0][0].numpy(), masks[0][0][1].numpy(), masks[0][0][2].numpy()]
+
+for i, mask in enumerate(mask_list, start=1):
+ overlayed_image = np.array(image).copy()
+
+ overlayed_image[:,:,0] = np.where(mask == 1, 255, overlayed_image[:,:,0])
+ overlayed_image[:,:,1] = np.where(mask == 1, 0, overlayed_image[:,:,1])
+ overlayed_image[:,:,2] = np.where(mask == 1, 0, overlayed_image[:,:,2])
+
+ axes[i].imshow(overlayed_image)
+ axes[i].set_title(f'Mask {i}')
+for ax in axes:
+ ax.axis('off')
+
+plt.show()
+```
+
+
+

+
+
+### Box Prompting
+
+You can also do box prompting in a similar fashion to point prompting. You can simply pass the input box in the format of a list
+`[x_min, y_min, x_max, y_max]` format along with the image to the `processor`. Take the processor output and directly pass it
+to the model, then post-process the output again.
+
+
+```python
+# bounding box around the bee
+box = [2350, 1600, 2850, 2100]
+
+inputs = processor(
+ image,
+ input_boxes=[[[box]]],
+ return_tensors="pt"
+ ).to("cuda")
+
+with torch.no_grad():
+ outputs = model(**inputs)
+
+mask = processor.image_processor.post_process_masks(
+ outputs.pred_masks.cpu(),
+ inputs["original_sizes"].cpu(),
+ inputs["reshaped_input_sizes"].cpu()
+)[0][0][0].numpy()
+```
+
+You can visualize the bounding box around the bee as shown below.
+
+```python
+import matplotlib.patches as patches
+
+fig, ax = plt.subplots()
+ax.imshow(image)
+
+rectangle = patches.Rectangle((2350, 1600, 500, 500, linewidth=2, edgecolor='r', facecolor='none')
+ax.add_patch(rectangle)
+ax.axis("off")
+plt.show()
+```
+
+
+

+
+
+You can see the inference output below.
+
+```python
+fig, ax = plt.subplots()
+ax.imshow(image)
+ax.imshow(mask, cmap='viridis', alpha=0.4)
+
+ax.axis("off")
+plt.show()
+```
+
+
+
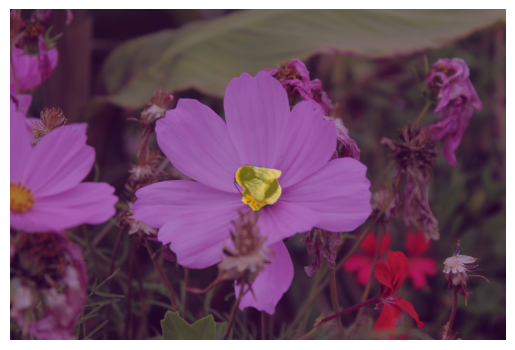
+
+